
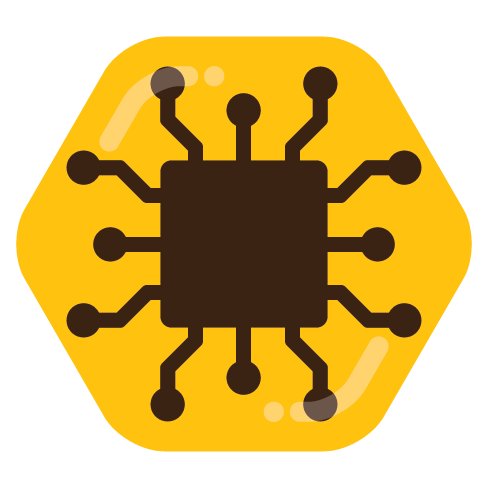
The problem is that while LLMs can translate, it’s still machine translation and isn’t always accurate. It’s also not going to just be for that. It’ll be applying “AI” to everything that looks like it might vaguely fit, and it’ll stifle productivity.
I feel like this article is exactly the type of thing it’s criticizing.